Contents
- 1 Section I: Long answer questions (word limit – 500 words). Each question carries 20 marks. Word limit does not apply in the case of numerical questions.
- 2 (a) Distinguish between the Population Regression Function and Sample Regression Function in detail. Use appropriate diagram to substantiate your response.
- 3 (b) What are the assumptions of a classical regression model?
- 4 2. (a) Measurement error in variables is a serious problem in econometric studies. Find out the consequences of measurement errors in i) dependent variable and ii) independent variables.
- 5 Section II: Medium answer questions (word limit – 250 words). Each question carries 10 marks. Word limit does not apply in the case of numerical questions
- 6 3. Differentiate between Chi-square distribution and t-distribution.
- 7 4. What is an estimator? Explain all the properties of an estimator with reference to BLUE.
- 8 5. Two variable regression model could have three functional forms as given below:𝑌i = 𝛽1 + 𝛽2𝑋i + 𝑢i𝑙𝑛𝑌i = 𝛽1 + 𝛽2𝑋i + 𝑢iln𝑌i = 𝛽1 + 𝛽2lnXi + 𝑢i
- 9 How will you decide which is the best model for a given econometric problem?
- 10 Section III: Short answer questions (word limit – 100 words). Each question carries 6 marks. Word limit does not apply in the case of numerical questions.
- 11 6. Discuss the remedial measures of multicollinearity.
- 12 7. What do we mean by Normal Distribution? Explain with the help of a diagram.
- 13 8. There are two types of estimation of parameters: Point Estimation and Interval Estimation. Explain the interval estimation method briefly.
- 14 9. What are the three methods of estimation? Discuss.
- 15 10. Explain the rejection regions for small samples and large samples.
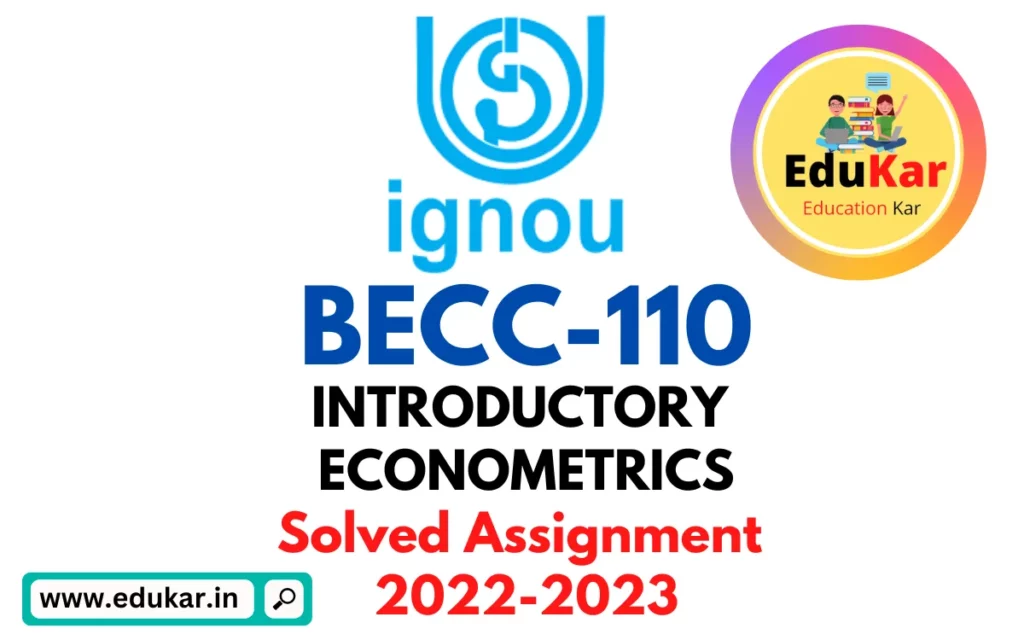
Title | BECC-110: IGNOU BAG Solved Assignment 2022-2023 |
University | IGNOU |
Degree | Bachelor Degree Programme |
Course Code | BECC-110 |
Course Name | INTRODUCTORY ECONOMETRICS |
Programme Name | Bachelor of Arts (General) |
Programme Code | BAG |
Total Marks | 100 |
Year | 2022-2023 |
Language | English |
Assignment Code | BECC-110/TMA /2022-23 |
Last Date for Submission of Assignment: | For June Examination: 31st April For December Examination: 30th September |
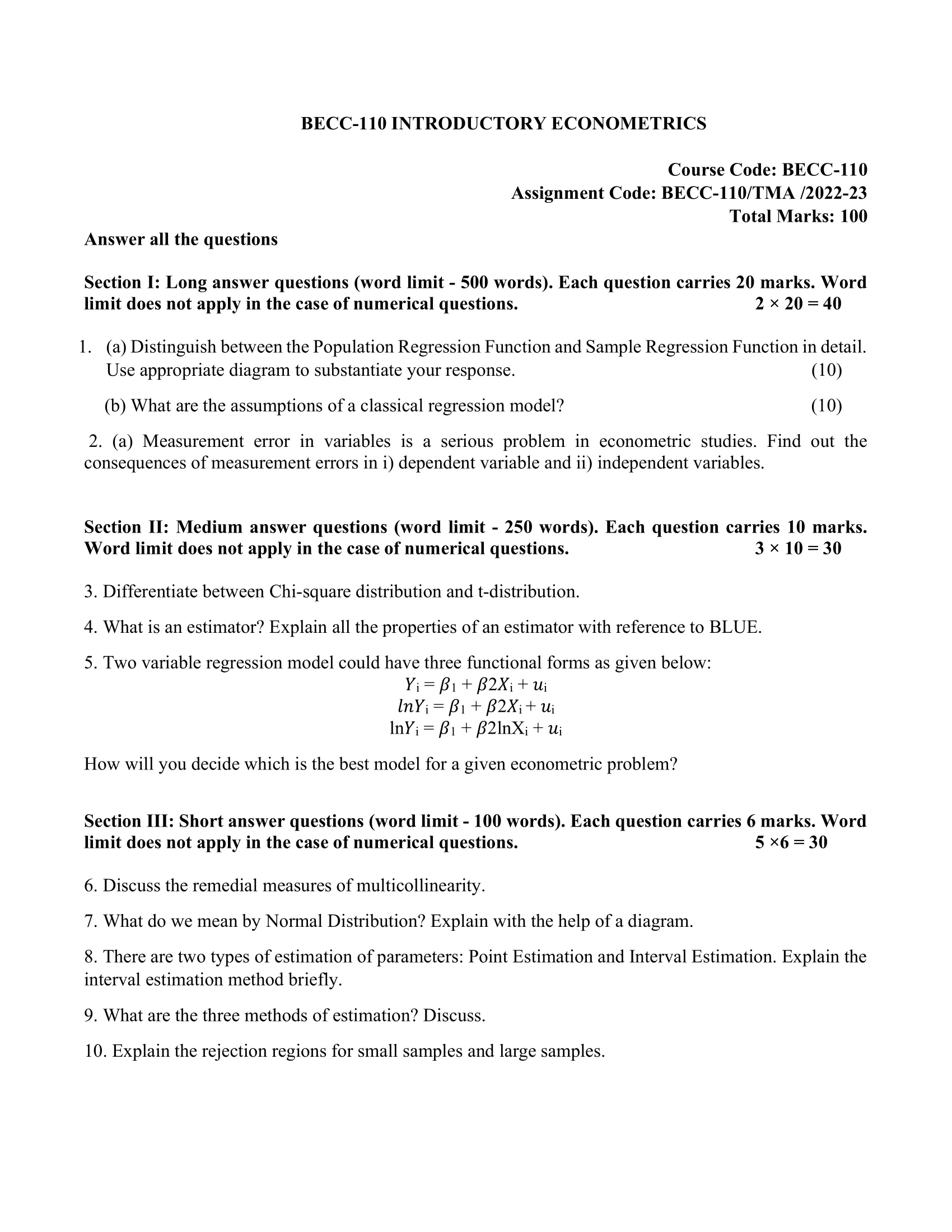
Section I: Long answer questions (word limit – 500 words). Each question carries 20 marks. Word limit does not apply in the case of numerical questions.
(a) Distinguish between the Population Regression Function and Sample Regression Function in detail. Use appropriate diagram to substantiate your response.
Ans: The population regression function and sample regression function are two statistical concepts used in regression analysis. They are used to describe the relationship between a dependent variable and one or more independent variables.
The population regression function (PRF) is a theoretical concept that represents the average relationship between the dependent variable and the independent variables for a population. It is defined as the expected value of the dependent variable for a given value of the independent variable(s). In other words, it represents the mean value of the dependent variable for a given value of the independent variable(s) in the population.
The sample regression function (SRF), on the other hand, is an estimate of the PRF based on a sample of the population. It is used to predict the value of the dependent variable for a given value of the independent variable(s) using the data in the sample. In other words, the SRF represents the relationship between the dependent and independent variables in the sample data, which can be used to make predictions about the population.
The difference between the PRF and SRF can be visualized in the form of a scatter plot. A scatter plot is a graphical representation of the relationship between two variables. In the case of regression analysis, the scatter plot represents the relationship between the dependent variable and the independent variable(s).
For example, consider a population of heights and weights of individuals. The PRF for this population would represent the mean height for a given weight in the population. The SRF, on the other hand, would represent the relationship between height and weight in the sample data, which would be an estimate of the PRF.
The PRF is typically represented by a smooth curve, while the SRF is represented by a line that passes through the sample data points. The SRF is a better estimate of the PRF when the sample size is large, and the sample data is representative of the population. However, when the sample size is small or the sample is not representative of the population, the SRF may deviate significantly from the PRF.
(b) What are the assumptions of a classical regression model?
Ans: Classical regression models make a set of assumptions about the data and the relationship between the dependent variable and independent variables. These assumptions are critical for obtaining accurate and meaningful results from a regression analysis. The most commonly used classical regression models are linear regression models and make the following assumptions:
- Linearity: The relationship between the dependent variable and independent variables is linear. This means that the change in the dependent variable is proportional to the change in the independent variable(s).
- Independence: The observations in the data are independent and not correlated with each other. This means that the value of the dependent variable for one observation is not influenced by the values of the dependent variable for other observations.
- Homoscedasticity: The variance of the errors (the difference between the observed dependent variable and the predicted dependent variable) is constant across all levels of the independent variable(s). This means that the spread of the errors is the same for all values of the independent variable(s).
- Normality: The errors are normally distributed. This means that the distribution of the errors is symmetric and bell-shaped.
- No multicollinearity: The independent variables are not highly correlated with each other. This means that two or more independent variables do not provide redundant information about the dependent variable.
- No autocorrelation: The errors are not autocorrelated. This means that the value of the error for one observation is not related to the value of the error for another observation.
These assumptions are important for the validity of the regression analysis and for making valid inferences about the population based on the sample data. It is important to assess the validity of these assumptions and make appropriate adjustments if necessary to ensure accurate results. Violations of these assumptions can lead to biased and unreliable results, so it is important to understand the limitations of classical regression models and choose the appropriate regression model for the data and the research question being addressed.
2. (a) Measurement error in variables is a serious problem in econometric studies. Find out the consequences of measurement errors in i) dependent variable and ii) independent variables.
Ans: Measurement error in variables can have significant consequences in econometric studies, particularly if the errors are systematic and not random.
- Consequences of measurement error in the dependent variable:
- Bias: If the measurement error in the dependent variable is not random, it can lead to biased estimates of the parameters in the regression model. This bias can result in incorrect inferences about the relationship between the independent variables and the dependent variable.
- Incorrect standard errors: Measurement error in the dependent variable can also lead to incorrect standard errors, which in turn can lead to incorrect inferences about the significance of the estimated parameters.
- Incorrect hypothesis tests: Measurement error in the dependent variable can also affect hypothesis tests, leading to incorrect decisions about the significance of the parameters and the validity of the model.
- Consequences of measurement error in the independent variables:
- Bias: Measurement error in the independent variables can also result in biased estimates of the parameters in the regression model. This can lead to incorrect inferences about the relationship between the dependent variable and the independent variables.
- Incorrect standard errors: Measurement error in the independent variables can also lead to incorrect standard errors, which in turn can lead to incorrect inferences about the significance of the estimated parameters.
- Incorrect hypothesis tests: Measurement error in the independent variables can also affect hypothesis tests, leading to incorrect decisions about the significance of the parameters and the validity of the model.
- Reduced precision: Measurement error in the independent variables can reduce the precision of the estimated parameters, making it more difficult to detect a significant relationship between the dependent variable and the independent variables.
Section II: Medium answer questions (word limit – 250 words). Each question carries 10 marks. Word limit does not apply in the case of numerical questions
3. Differentiate between Chi-square distribution and t-distribution.
Ans: Chi-square distribution and t-distribution are two different statistical distributions used in hypothesis testing and estimation.
The chi-square distribution is used to determine if there is a significant difference between an observed frequency distribution and a theoretical expected frequency distribution. It is a continuous probability distribution that is used to test the goodness-of-fit of an observed data set to a theoretical model. The chi-square distribution is characterized by its degrees of freedom, which is equal to the number of independent values in the data set minus one.
On the other hand, the t-distribution is used in inferential statistics when the sample size is small and the population standard deviation is unknown. It is a type of continuous probability distribution that resembles the standard normal distribution, but with slightly heavier tails. The shape of the t-distribution is determined by its degrees of freedom, which is equal to the sample size minus one.
One key difference between the two distributions is that the t-distribution is used to estimate population parameters when the sample size is small, whereas the chi-square distribution is used to test the goodness-of-fit of a data set to a theoretical model. Additionally, the t-distribution is used to calculate the t-statistic, which is used to test the hypothesis that the mean of a population is equal to a specified value.
4. What is an estimator? Explain all the properties of an estimator with reference to BLUE.
Ans: An estimator is a statistical method or formula used to calculate an estimate of a population parameter based on sample data. In other words, it is a statistical tool that takes a sample of data and provides an estimate of the value of an unknown population parameter.
There are several important properties of an estimator, and a good estimator is often referred to as an “unbiased estimator”. In particular, the best linear unbiased estimator (BLUE) is a widely used concept in statistics that is used to evaluate the properties of an estimator.
The properties of a BLUE estimator are as follows:
- Unbiasedness: An estimator is considered unbiased if its expected value is equal to the true population parameter being estimated. In other words, the average of the estimator’s values over many samples is equal to the true population parameter.
- Linearity: A BLUE estimator is a linear combination of the sample data, which means that the estimator can be expressed as a linear equation.
- Best: A BLUE estimator is considered the “best” estimator among all linear unbiased estimators, in the sense that it has the smallest variance of all linear unbiased estimators for the same sample size.
- Efficiency: A BLUE estimator is considered efficient because it has the smallest variance among all unbiased estimators. In other words, it provides the most accurate estimate of the population parameter.
5. Two variable regression model could have three functional forms as given below:
𝑌i = 𝛽1 + 𝛽2𝑋i + 𝑢i
𝑙𝑛𝑌i = 𝛽1 + 𝛽2𝑋i + 𝑢i
ln𝑌i = 𝛽1 + 𝛽2lnXi + 𝑢i
How will you decide which is the best model for a given econometric problem?
Ans: The choice of the best functional form for a two-variable regression model in econometrics depends on several factors, including the nature of the data and the underlying relationship between the independent and dependent variables. The following are some general guidelines that can help you decide which model is best for a given econometric problem:
- Linearity: If the relationship between the independent and dependent variables is linear, then the first functional form (𝑌i = 𝛽1 + 𝛽2𝑋i + 𝑢i) is a good choice. If the relationship is not linear, then the other two functional forms (𝑙𝑛𝑌i = 𝛽1 + 𝛽2𝑋i + 𝑢i and ln𝑌i = 𝛽1 + 𝛽2lnXi + 𝑢i) should be considered.
- Log-linear relationship: If the relationship between the independent and dependent variables is log-linear, then the second functional form (𝑙𝑛𝑌i = 𝛽1 + 𝛽2𝑋i + 𝑢i) is a good choice. This form is often used in economics and finance to model the relationship between the logarithm of two variables.
- Log-log relationship: If the relationship between the independent and dependent variables is log-log, then the third functional form (ln𝑌i = 𝛽1 + 𝛽2lnXi + 𝑢i) is a good choice. This form is often used in economics and finance to model the relationship between the logarithm of both the dependent and independent variables.
- Econometric theory: If the econometric problem has a well-established theoretical framework, it is important to consider the theoretical predictions when choosing the functional form. For example, if the theory predicts a log-linear relationship, then the second functional form should be considered.
Section III: Short answer questions (word limit – 100 words). Each question carries 6 marks. Word limit does not apply in the case of numerical questions.
6. Discuss the remedial measures of multicollinearity.
Ans: Multicollinearity is a common problem in regression analysis, where two or more independent variables are highly correlated with each other. The following are some remedial measures for multicollinearity:
- Variable selection: Removing one or more of the highly correlated independent variables can help reduce the multicollinearity.
- Transformation: Transforming the independent variables, such as taking the logarithm or square root of the variables, can reduce the multicollinearity.
- Regularization methods: Methods such as ridge regression and lasso can be used to address multicollinearity by adding a penalty term to the regression analysis to reduce the magnitude of the estimated coefficients.
- Principal component analysis: This method involves transforming the independent variables into a set of new variables that are uncorrelated with each other and can help reduce the multicollinearity.
- Collinearity diagnostics: Conducting a collinearity diagnostics, such as calculating the variance inflation factor (VIF), can help identify the magnitude of the multicollinearity and suggest appropriate remedial measures.
It is important to address multicollinearity in regression analysis as it can lead to unstable and unreliable estimates of the parameters and can affect the validity of hypothesis tests and inferences about the relationships between the variables.
7. What do we mean by Normal Distribution? Explain with the help of a diagram.
Ans: The normal distribution, also known as the Gaussian distribution or bell curve, is a continuous probability distribution that is symmetrical about the mean. It is one of the most widely used and well-known distributions in statistics and is commonly used to model various continuous variables such as height, weight, IQ, and test scores.
In a normal distribution, the majority of the observations are clustered around the mean, with fewer observations as you move further away from the mean. The spread of the distribution is determined by the standard deviation, which measures the degree of variability around the mean.
A diagram can help to visualize the normal distribution. In a normal distribution, the horizontal axis represents the variable of interest (e.g. height), and the vertical axis represents the frequency of the observations. The distribution is shaped like a bell, with the highest point at the mean and a gradual decrease in frequency as you move away from the mean in either direction. The standard deviation determines the width of the bell, with a larger standard deviation resulting in a wider bell and vice versa.
It is important to note that many variables in real-life are not perfectly normally distributed, but the normal distribution is still often used as a model for continuous variables because of its simplicity and tractability. In such cases, the normality assumption can be tested using various statistical tests such as the Shapiro-Wilk test or the Anderson-Darling test.
8. There are two types of estimation of parameters: Point Estimation and Interval Estimation. Explain the interval estimation method briefly.
Ans: Interval estimation is a method of estimating the value of a population parameter by constructing an interval around a point estimate that contains the true value of the parameter with a specified degree of confidence. The interval is referred to as a confidence interval, and the specified degree of confidence is called the confidence level.
The main idea behind interval estimation is that if we were to repeat the estimation process many times using different samples, the resulting intervals would contain the true value of the parameter a specified percentage of the time. For example, if the confidence level is 95%, the confidence interval would contain the true value of the parameter 95% of the time if the estimation process were repeated many times.
To construct a confidence interval, we first estimate the population parameter using a point estimate, such as the sample mean or sample proportion. We then use statistical methods, such as the t-distribution or the normal distribution, to calculate the margin of error around the point estimate. This margin of error is added and subtracted from the point estimate to obtain the upper and lower bounds of the confidence interval.
Interval estimation provides more information about the uncertainty of the estimation process than point estimation alone, as it provides a range of values that are likely to contain the true value of the parameter with a specified degree of confidence. This allows us to make more informed decisions and draw more robust conclusions about the relationships between variables in our data.
9. What are the three methods of estimation? Discuss.
Ans: There are three main methods of estimation in statistics:
- Point estimation: Point estimation is the process of finding a single value that is representative of the population parameter. The point estimate is usually obtained using a sample statistic, such as the sample mean or sample proportion. The main disadvantage of point estimation is that it provides limited information about the uncertainty of the estimate and does not account for the variability in the data.
- Interval estimation: Interval estimation is the process of constructing an interval around a point estimate that contains the true value of the population parameter with a specified degree of confidence. Interval estimation provides more information about the uncertainty of the estimate compared to point estimation, as it provides a range of values that are likely to contain the true value of the population parameter.
- Bayesian estimation: Bayesian estimation is a statistical approach that uses prior information about the population parameter to update our understanding of the parameter based on new data. Bayesian estimation is based on Bayes’ theorem, which states that the probability of a hypothesis given the data is proportional to the prior probability of the hypothesis multiplied by the likelihood of the data given the hypothesis. Bayesian estimation provides a way to incorporate prior knowledge into the estimation process and can lead to more accurate estimates in some situations compared to other methods.
Each of these methods has its own strengths and weaknesses, and the choice of method depends on the problem at hand, the nature of the data, and the goals of the estimation. In many cases, point estimation and interval estimation are sufficient for making inferences about the population, while Bayesian estimation is used in specialized applications where prior information about the population parameter is available and needs to be incorporated into the estimation process.
10. Explain the rejection regions for small samples and large samples.
Ans: Rejection regions are areas in a hypothesis test where the null hypothesis is rejected in favor of the alternative hypothesis. The rejection region is determined by the level of significance of the test, and it is based on the distribution of the test statistic under the null hypothesis.
For small samples, the rejection region is based on the t-distribution, which takes into account the uncertainty in estimating the population mean and variance from a small sample. The t-distribution becomes more spread out as the sample size decreases, so the rejection region is larger for smaller sample sizes.
For large samples, the rejection region is based on the normal distribution, which is a good approximation to the t-distribution as the sample size increases. In this case, the rejection region is determined by the critical values of the standard normal distribution. In general, the rejection region is smaller for larger sample sizes, as the normal distribution is less spread out than the t-distribution.
How to Download BECC-110 Solved Assignment?
You can download it from the www.edukar.in, they have a big database for all the IGNOU solved assignments.
Is the BECC-110 Solved Assignment Free?
Yes this is absolutely free to download the solved assignment from www.edukar.in
What is the last submission date for BECC-110 Solved Assignment?
For June Examination: 31st April, For December Examination: 30th October