Contents
- 1 A. Long Answer Questions (word limit-500 words) 2 × 20 = 40 marks
- 2 1) Explain, theoretically, the consequences of omitting relevant variables in econometric modelling.
- 3 2) Discuss the effect of lags on ‘market equilibrium’ with suitable examples.
- 4 B. Medium Answer Questions (word limit-250 words) 3 × 10 = 30 marks
- 5 3) Outline how R2 and adjusted-R2 serve as indicators of ‘goodness of fit’ of a regression model.
- 6 4) Indicate the form of a Logit Model. Specify why the OLS method of estimation cannot be applied here.
- 7 5) Show that ‘exact identification’ is a sufficient condition for the unique determinationof a system of equations.
- 8 C. Short Answer Questions (word limit 100 words) 2 × 3 × 5 = 30 marks
- 9 6) Differentiate between:(a) Quantitative Research and Qualitative Research.
- 10 (b) In-sample forecast and out-of-sample forecast.
- 11 (c) Autoregressive Model and Autoregressive Distributed Lag Model.
- 12 7) Write short notes on the following.
- 13 (a) Instrumental Variables (IV) Method.
- 14 (b) Pooled Cross Section Data.
- 15 (c) Linear Static Panel Data Model.
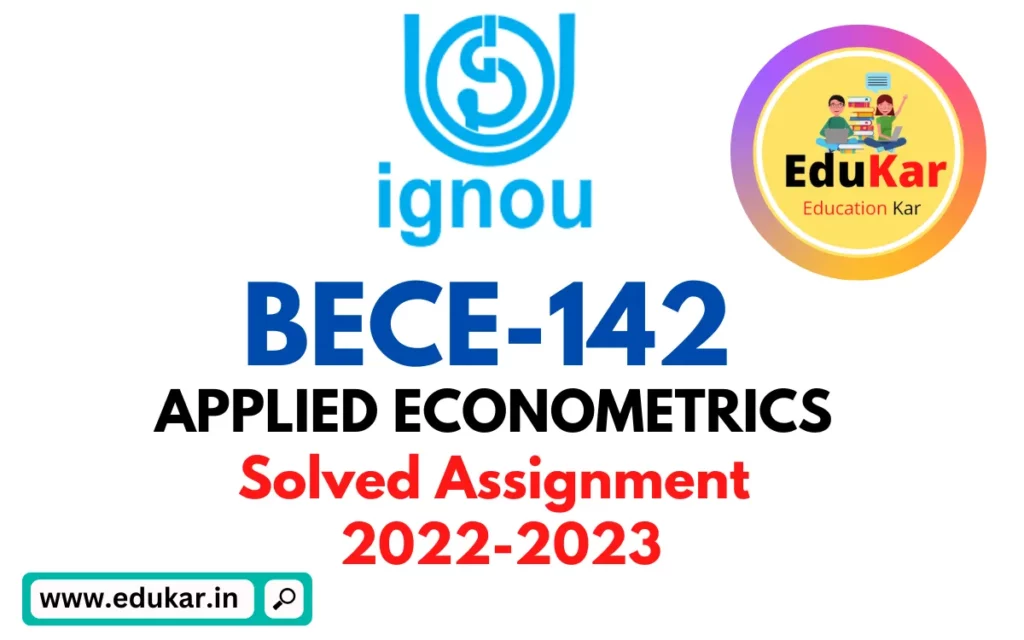
Title | BECE-142: IGNOU BAG Solved Assignment 2022-2023 |
University | IGNOU |
Degree | Bachelor Degree Programme |
Course Code | BECE-142 |
Course Name | APPLIED ECONOMETRICS |
Programme Name | Bachelor of Arts (General) |
Programme Code | BAG |
Total Marks | 100 |
Year | 2022-2023 |
Language | English |
Assignment Code | BECE-142/AST/TMA/2022-23 |
Last Date for Submission of Assignment: | For June Examination: 31st April For December Examination: 30th September |
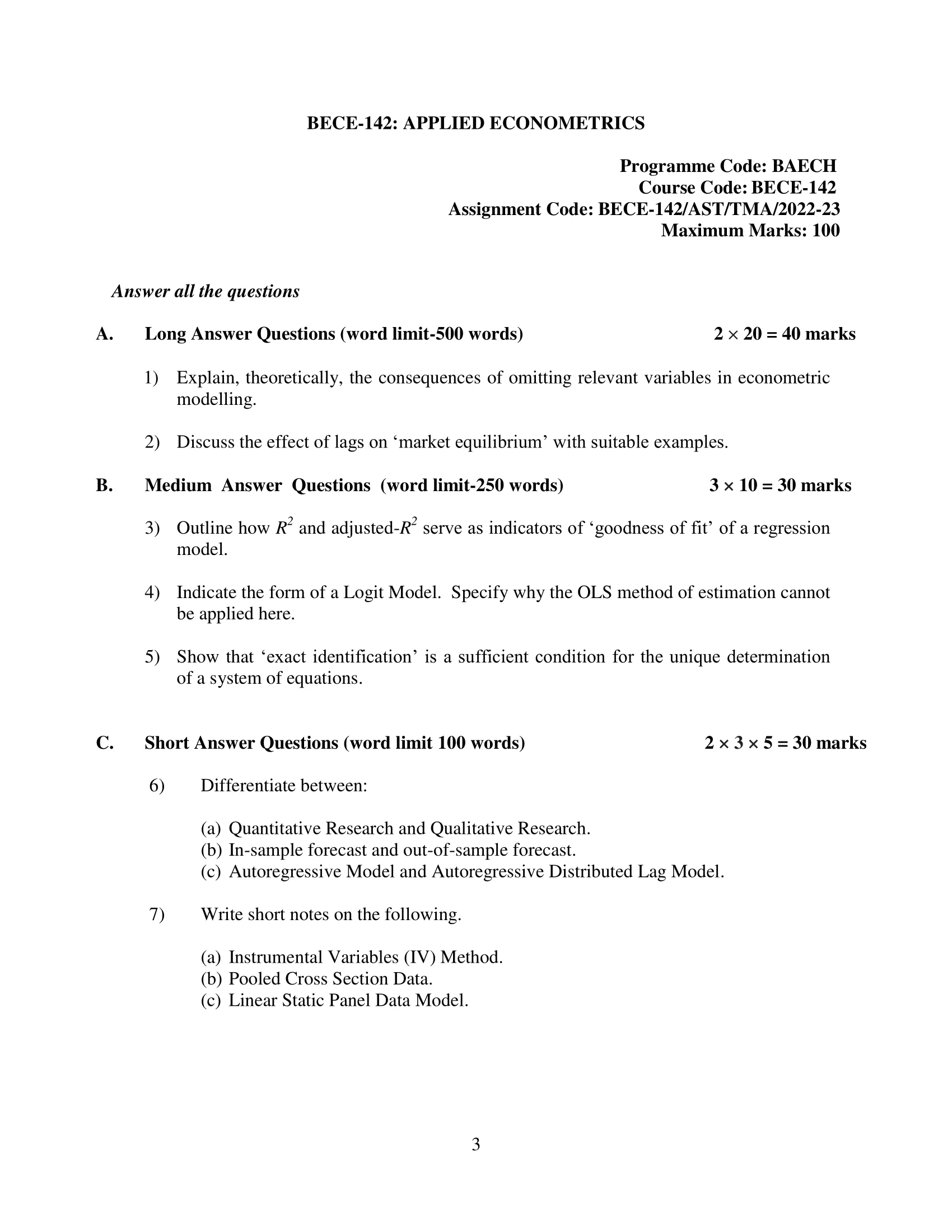
A. Long Answer Questions (word limit-500 words) 2 × 20 = 40 marks
1) Explain, theoretically, the consequences of omitting relevant variables in econometric modelling.
Ans: In econometric modeling, the goal is to establish a relationship between a dependent variable and one or more independent variables. This relationship is usually represented in the form of a statistical model. Omitting relevant variables in econometric modeling can have serious consequences, both theoretically and practically.
Theoretically, omitting relevant variables leads to a biased estimate of the relationship between the dependent and independent variables. This bias can be in the form of omitted variable bias, which occurs when the omitted variable is correlated with both the dependent variable and one of the independent variables. In this case, the omitted variable will affect the estimate of the relationship between the dependent and independent variables.
For example, consider a simple linear regression model where the dependent variable is wage and the independent variable is education. If we omit the variable experience, which is likely to be correlated with both wage and education, the estimate of the effect of education on wage may be biased.
The bias in the estimate of the relationship between the dependent and independent variables can lead to incorrect inferences and conclusions. For example, if the omitted variable is positively correlated with the dependent variable and negatively correlated with one of the independent variables, the estimate of the relationship between the dependent and independent variables may indicate that the independent variable has a negative effect on the dependent variable, when in reality it has a positive effect.
In addition to the theoretical consequences, omitting relevant variables in econometric modeling can also have practical consequences. For example, if the omitted variable is important in explaining the variation in the dependent variable, the model will have poor predictive power. This means that the model may not be able to accurately predict the value of the dependent variable, which is particularly important in policy-making and decision-making.
Another practical consequence of omitting relevant variables is that it can lead to the use of incorrect or suboptimal policies. For example, if a model is used to inform policy decisions, such as setting a minimum wage, and the model omits important variables such as experience or regional differences, the policy may have unintended consequences, such as reducing employment or migration.
2) Discuss the effect of lags on ‘market equilibrium’ with suitable examples.
Ans: In economics, market equilibrium refers to a state where the quantity of a good or service supplied is equal to the quantity demanded. This results in a stable market price that remains unchanged until a shift in either supply or demand occurs. The effect of lags on market equilibrium can be significant and can result in changes in market prices, quantities, and the overall level of economic activity.
Lags refer to the time it takes for a change in one variable to have an effect on another variable. For example, a change in the price of a good can take time to affect the quantity demanded, and a change in the quantity of a good supplied can take time to affect the price. In the context of market equilibrium, lags can cause changes in supply or demand to have delayed effects on market prices and quantities.
For example, consider a market for a new product, such as a smartphone. A sudden increase in demand for the smartphone may cause a temporary shortage and an increase in the market price. However, as suppliers respond to the increased demand, they may take some time to ramp up production, leading to a lag in the increase in supply. This lag in supply can result in a temporary overshoot in the market price, as the quantity supplied is still lower than the quantity demanded.
Another example of the effect of lags on market equilibrium is the impact of monetary policy on the economy. A change in interest rates can take time to affect the level of economic activity, as consumers and businesses adjust their spending and investment decisions. This lag can result in a delay in the effects of monetary policy on the economy, causing the market to temporarily overshoot or undershoot its equilibrium.
Lags can also have an impact on market clearing, which refers to the point at which the quantity supplied is equal to the quantity demanded. For example, in a market with a long lag in the adjustment of supply, the market may not clear immediately following a shift in demand, leading to a temporary surplus or shortage of the good or service.
In addition to the effects of lags on market prices and quantities, they can also impact the overall level of economic activity. For example, a lag in the response of suppliers to changes in demand can result in fluctuations in the level of production and employment, as suppliers ramp up or down production in response to changes in demand.
B. Medium Answer Questions (word limit-250 words) 3 × 10 = 30 marks
3) Outline how R2 and adjusted-R2 serve as indicators of ‘goodness of fit’ of a regression model.
Ans: R2 and adjusted R2 are measures used to evaluate the goodness of fit of a regression model. These metrics indicate how well the model fits the data, and they help to determine whether the model is a good representation of the underlying relationship between the independent and dependent variables.
R2, also known as the coefficient of determination, is the proportion of the variance in the dependent variable that is explained by the model. A high R2 value means that the model is a good fit for the data, as it is explaining a large portion of the variation in the dependent variable. An R2 value close to 1 indicates that the model is a very good fit, while an R2 value close to 0 means that the model is a poor fit for the data.
However, R2 has some limitations, particularly when the number of independent variables increases. In this situation, R2 may become artificially high, even if the model is a poor fit for the data. To address this issue, the adjusted R2 was introduced.
Adjusted R2 is a modification of R2 that adjusts for the number of independent variables in the model. The adjusted R2 will always be lower than the R2 value, but it provides a more accurate representation of the model’s fit. A high adjusted R2 value indicates that the model is a good fit for the data, while a low adjusted R2 value means that the model is a poor fit.
4) Indicate the form of a Logit Model. Specify why the OLS method of estimation cannot be applied here.
Ans: A logit model is a type of regression model used in statistics and economics to model binary dependent variables. The form of a logit model is as follows:
logit(p) = β0 + β1×1 + β2×2 + … + βkxk
where p is the probability of the binary dependent variable (such as success or failure), and x1, x2, …, xk are the independent variables. The coefficients β0, β1, β2, …, βk are estimated using maximum likelihood estimation techniques.
The OLS (Ordinary Least Squares) method of estimation cannot be applied to logit models because the OLS method assumes that the dependent variable is continuous and normally distributed. However, binary dependent variables have a limited range of values (0 or 1) and are not normally distributed. The logit transformation of the dependent variable results in a logistic distribution, which is more appropriate for modeling binary dependent variables.
Another reason why OLS cannot be applied to logit models is that the dependent variable in a logit model is a probability, which must be between 0 and 1. OLS estimates do not guarantee that the predicted probabilities will fall within this range. The logit model, on the other hand, ensures that the predicted probabilities fall within the range of 0 and 1 by transforming the dependent variable into log odds.
5) Show that ‘exact identification’ is a sufficient condition for the unique determination
of a system of equations.
Ans: Exact identification refers to a situation in which the number of parameters in a system of equations is equal to the number of observables. In other words, the system of equations contains enough information to uniquely determine the values of the parameters. When exact identification is satisfied, the system of equations can be solved for the parameters without any ambiguity.
A sufficient condition for the unique determination of a system of equations is the existence of a unique solution. This means that there is only one set of parameter values that satisfies the system of equations. In other words, there is no degeneracy or redundancy in the information contained in the system of equations.
Exact identification is a sufficient condition for the unique determination of a system of equations because it ensures that the number of parameters is equal to the number of observables. This means that the system of equations contains enough information to uniquely determine the values of the parameters. As a result, there is only one set of parameter values that satisfies the system of equations, and the system can be solved for the parameters without any ambiguity.
C. Short Answer Questions (word limit 100 words) 2 × 3 × 5 = 30 marks
6) Differentiate between:
(a) Quantitative Research and Qualitative Research.
Ans: Quantitative research and qualitative research are two distinct methods of data collection and analysis in the social sciences. Quantitative research is based on numerical data and statistical analysis and is often used to test hypotheses and make predictions. This type of research is typically structured and focuses on finding cause-and-effect relationships between variables. On the other hand, qualitative research is based on non-numerical data, such as interviews, observations, and texts, and is often used to gain an in-depth understanding of a particular phenomenon. This type of research is more exploratory in nature and focuses on the interpretation of meaning and understanding subjective experiences.
(b) In-sample forecast and out-of-sample forecast.
Ans: In-sample forecast and out-of-sample forecast are two types of forecasting methods used in economics and finance. An in-sample forecast uses data that was used to build the model to make predictions. The model is developed and tested on the same data set, which is used to make predictions. The in-sample forecast is considered to be less reliable than the out-of-sample forecast as it may not be representative of the data and may result in overfitting.
On the other hand, an out-of-sample forecast uses data that was not used to build the model to make predictions. The model is developed using a portion of the data and then tested on the remaining portion of the data. The out-of-sample forecast is considered to be more reliable as it tests the model’s ability to make predictions using data it has not seen before. This method is commonly used to assess the accuracy of a model’s predictions and to validate the stability of the model’s parameters.
(c) Autoregressive Model and Autoregressive Distributed Lag Model.
Ans: Autoregressive (AR) model and Autoregressive Distributed Lag (ADL) model are two popular time series forecasting models in econometrics.
An Autoregressive model is a type of time series model that uses past values of a variable to predict its future values. The model assumes that the current value of a variable is a linear combination of its past values and a random error term. The order of the AR model, denoted as “p,” refers to the number of past values used in the model. For example, an AR(1) model uses only the last period’s value, while an AR(2) model uses the last two periods’ values, and so on.
On the other hand, an Autoregressive Distributed Lag model is a type of time series model that includes both past values and lagged values of other variables as explanatory variables. The model takes into account the dynamic inter-relationships between the variable of interest and other related variables, and uses this information to make predictions. The ADL model is often used when there are multiple interrelated variables in a time series, and the relationships between these variables change over time.
7) Write short notes on the following.
(a) Instrumental Variables (IV) Method.
Ans: The Instrumental Variables (IV) method is a statistical technique used in econometrics to estimate causal relationships between variables. It is often used when there is endogeneity, meaning that one of the independent variables is correlated with the error term. The IV method involves introducing an additional variable, called an instrumental variable, that is correlated with the independent variable but not with the error term. The instrumental variable is used to obtain an unbiased estimate of the causal relationship between the independent and dependent variables. The IV method can be used to estimate models with binary, continuous, or censored dependent variables, and it is widely used in applied microeconometric research.
(b) Pooled Cross Section Data.
Ans: Pooled cross-section data refers to data that consists of observations from multiple individuals or units (such as households, firms, or countries) at different points in time. The observations are combined into a single dataset, and the variation across units and over time is analyzed. Pooled cross-section data is commonly used in economics and social sciences to study how variables, such as income, consumption, or employment, change over time. The advantage of using pooled cross-section data is that it provides a larger sample size and allows for the analysis of the relationship between variables over time. However, it also has some limitations, such as the assumption that the relationships between variables are constant over time and the lack of information on individual units.
(c) Linear Static Panel Data Model.
Ans: The Linear Static Panel Data Model is a type of econometric model used to analyze panel data, or data that includes observations on multiple individuals or units (such as households, firms, or countries) over time. The model assumes that the relationships between variables are constant over time, and that the variables can be described by a linear equation. The model takes the form of:
y[i,t] = β0 + β1×1[i,t] + β2×2[i,t] + … + βkxk[i,t] + ε[i,t]
where y[i,t] is the dependent variable for individual i at time t, x1[i,t], x2[i,t], …, xk[i,t] are the independent variables, β0, β1, β2, …, βk are the coefficients, and ε[i,t] is the error term. The coefficients are estimated using least squares or maximum likelihood methods.
The Linear Static Panel Data Model is widely used in applied microeconometrics and is useful for analyzing the relationship between variables over time while controlling for individual heterogeneity. However, it has some limitations, such as the assumption of constant relationships over time and the need for a large sample size to obtain reliable estimates. In addition, the model may not be appropriate for analyzing dynamic relationships or for data with a high degree of individual heterogeneity.
How to Download BECE-142 Solved Assignment?
You can download it from the www.edukar.in, they have a big database for all the IGNOU solved assignments.
Is the BECE-142 Solved Assignment Free?
Yes this is absolutely free to download the solved assignment from www.edukar.in
What is the last submission date for BECE-142 Assignment?
For June Examination: 31st April, For December Examination: 30th October